Figure 1. Two concepts of medicine
Science is the process of observing and interpreting reality to gain knowledge. This knowledge is then applied to technology. In the past, medicine has focused on the symptoms of disease, interpreted them based on mechanisms, and found many causes for the symptoms. In the future, medicine must observe and interpret the process by which signs of disease appear, disease progresses, and symptoms appear. At this point, mechanism-based interpretation cannot be used. This is because disease emerges through a bidirectional dynamic process in which the global order emerges bottom-up through local interactions among system components and between components and the environment, and the global order thus created governs the local interactions among components top-down as a boundary condition. By understanding this emergent process, it is possible to get to the underlying cause of disease.
From: Medical AI and AI for Medical Sciences
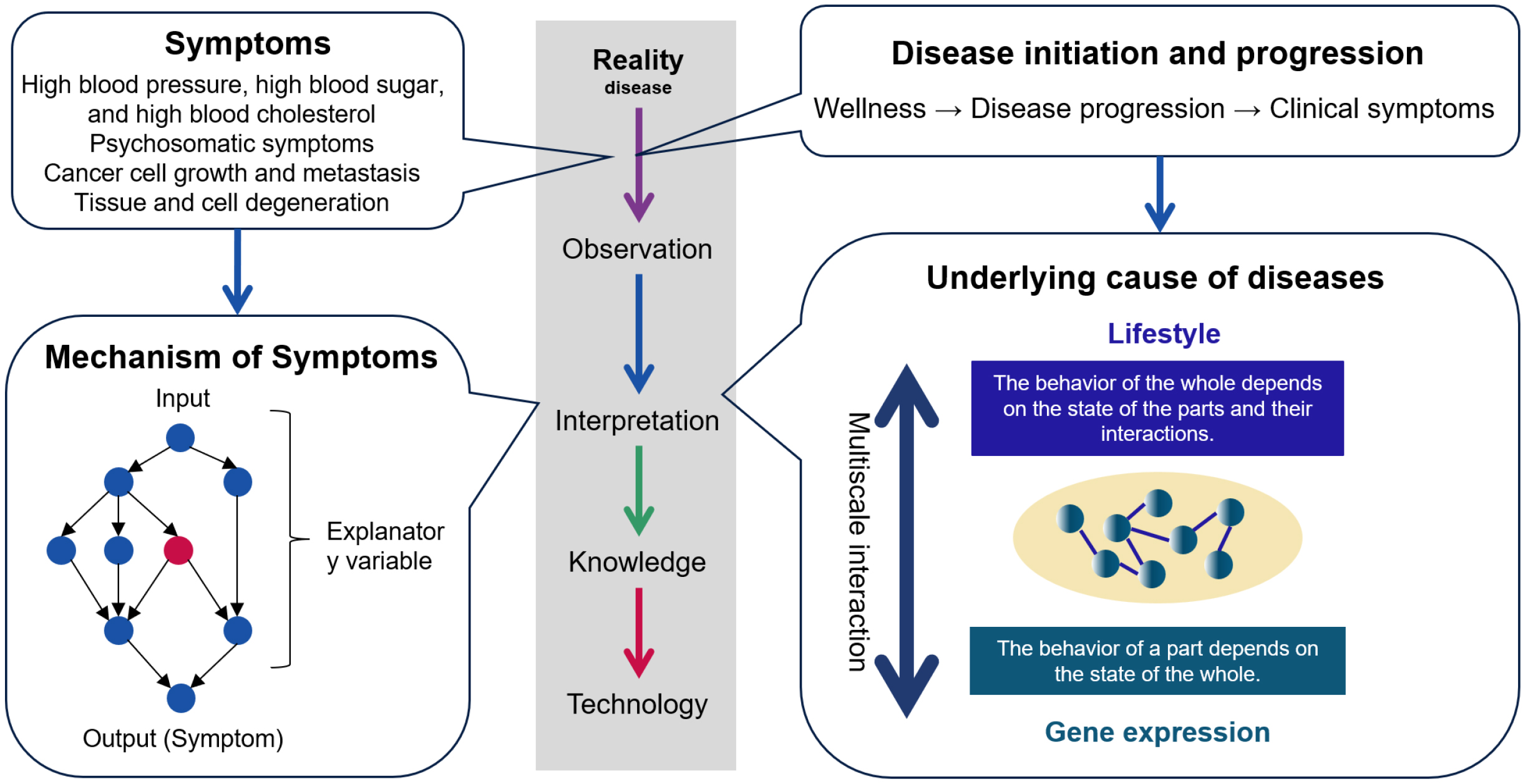
Figure 2. Analysis flow of ML
Feature vectors are obtained from a large number of test data representing patient characteristics. At this point, feature engineering is performed. ML uses the feature vector as input (x) to produce the output (y). Supervised learning aims to reproduce predetermined outputs, whereas unsupervised learning extracts a hidden pattern from the feature vector.
From: Medical AI and AI for Medical Sciences
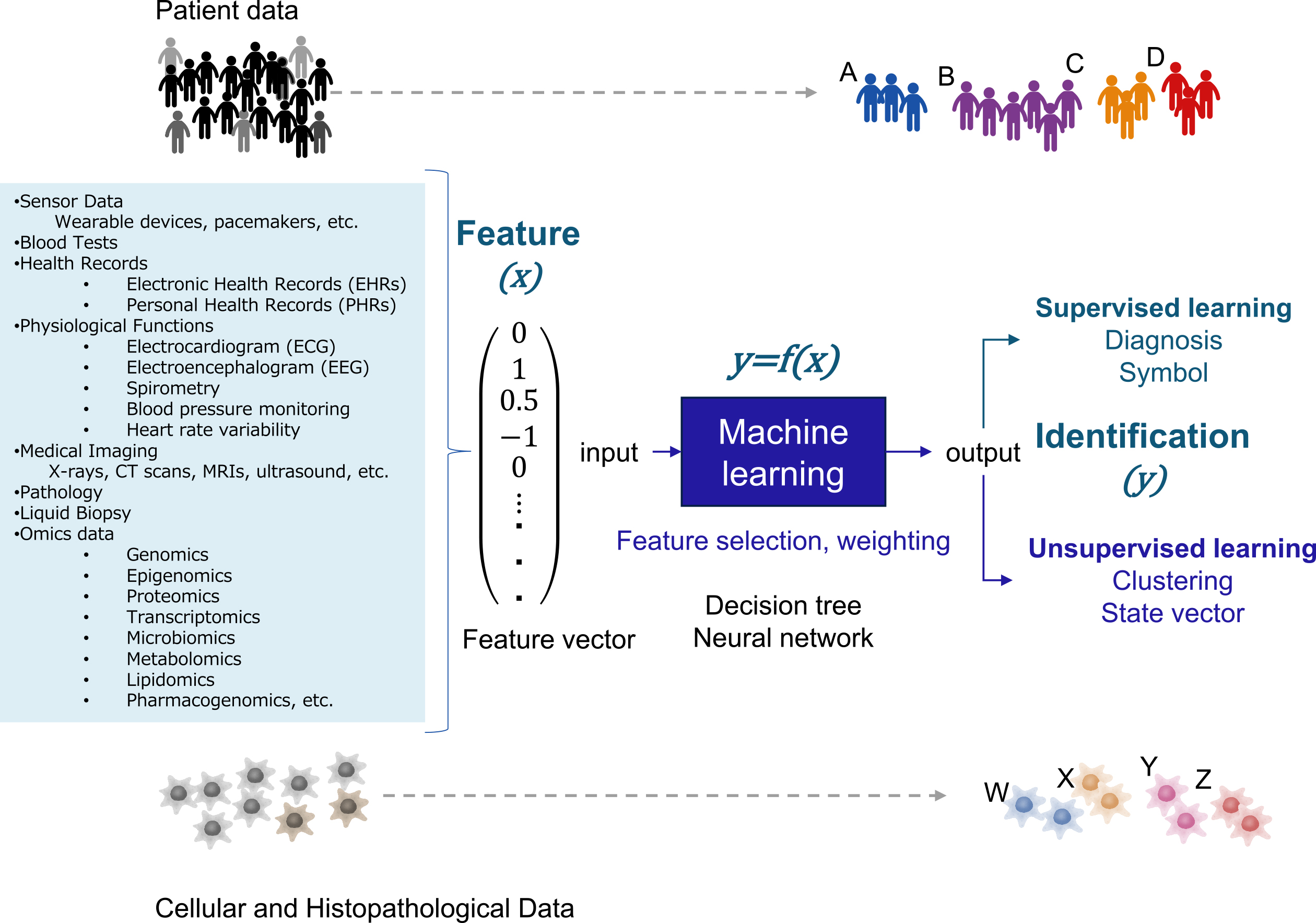
Figure 3. Development flow of a general-purpose medical AI model
Consider the case of developing a general-purpose medical AI model using an LLM. In this case, medical domain knowledge and clinical data are used as training data. The general-purpose medical AI produced in this way can be applied to bedside decision support, medical procedure augmentation, radiology reporting, interactive note taking, and patient chatbots.
From: Medical AI and AI for Medical Sciences
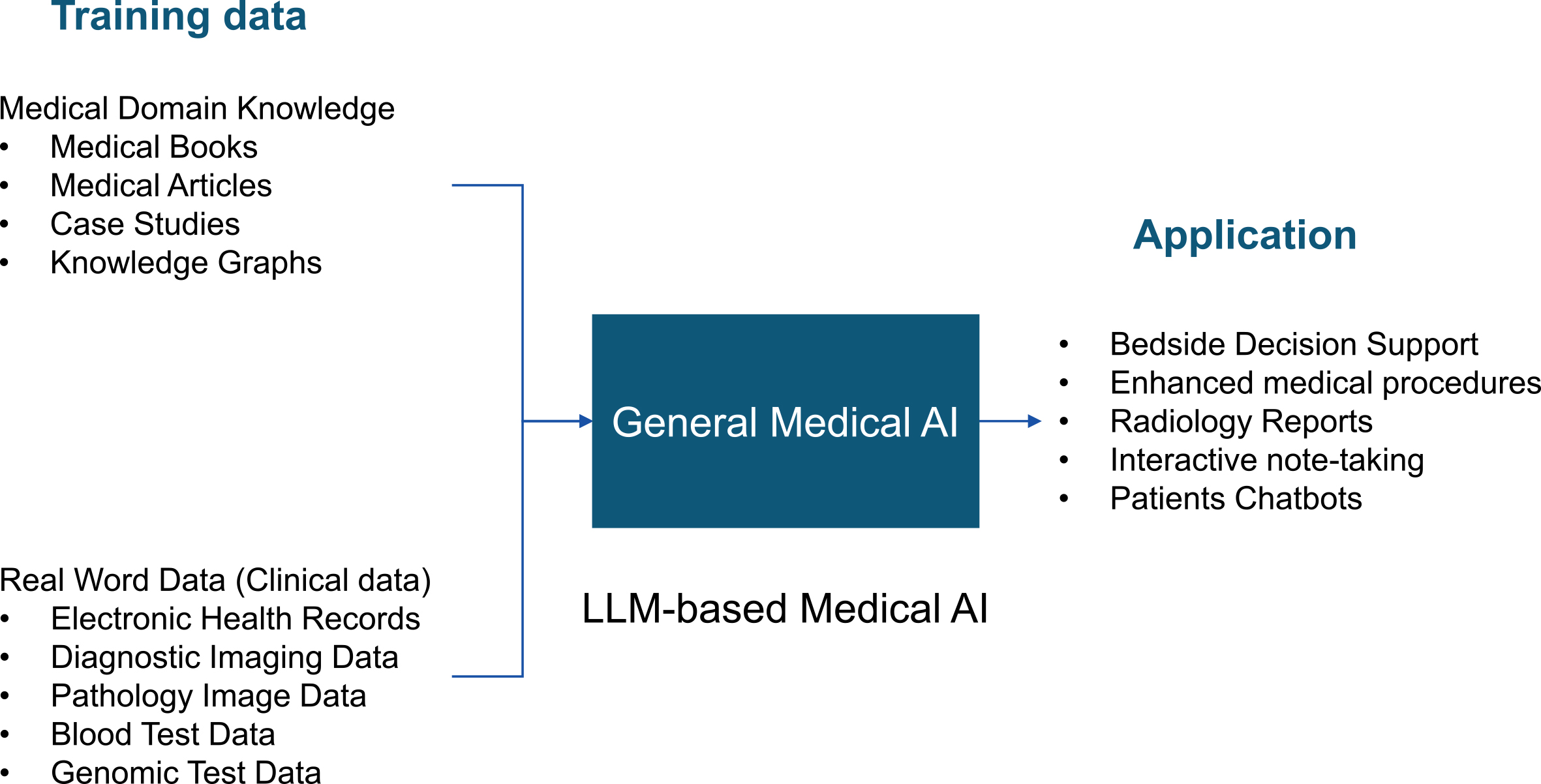
Figure 4. Scientific advances
Science was pioneered by empirical science (first generation), represented by medicine and biology, and theoretical science (second generation), represented by physics and chemistry. These two paradigms were applied to computational science (third generation) as the capabilities of computers improved. By contrast, the progress of digital transformation has led to the collection and integration of large amounts of real-world data. Data-intensive science (fourth generation), which uses AI and ML to analyze this data, was pioneered. Today, science-based AI (fifth generation) is being developed, which incorporates knowledge from empirical science and principles from theoretical science into the surrogate models developed in data-intensive science.
From: Medical AI and AI for Medical Sciences
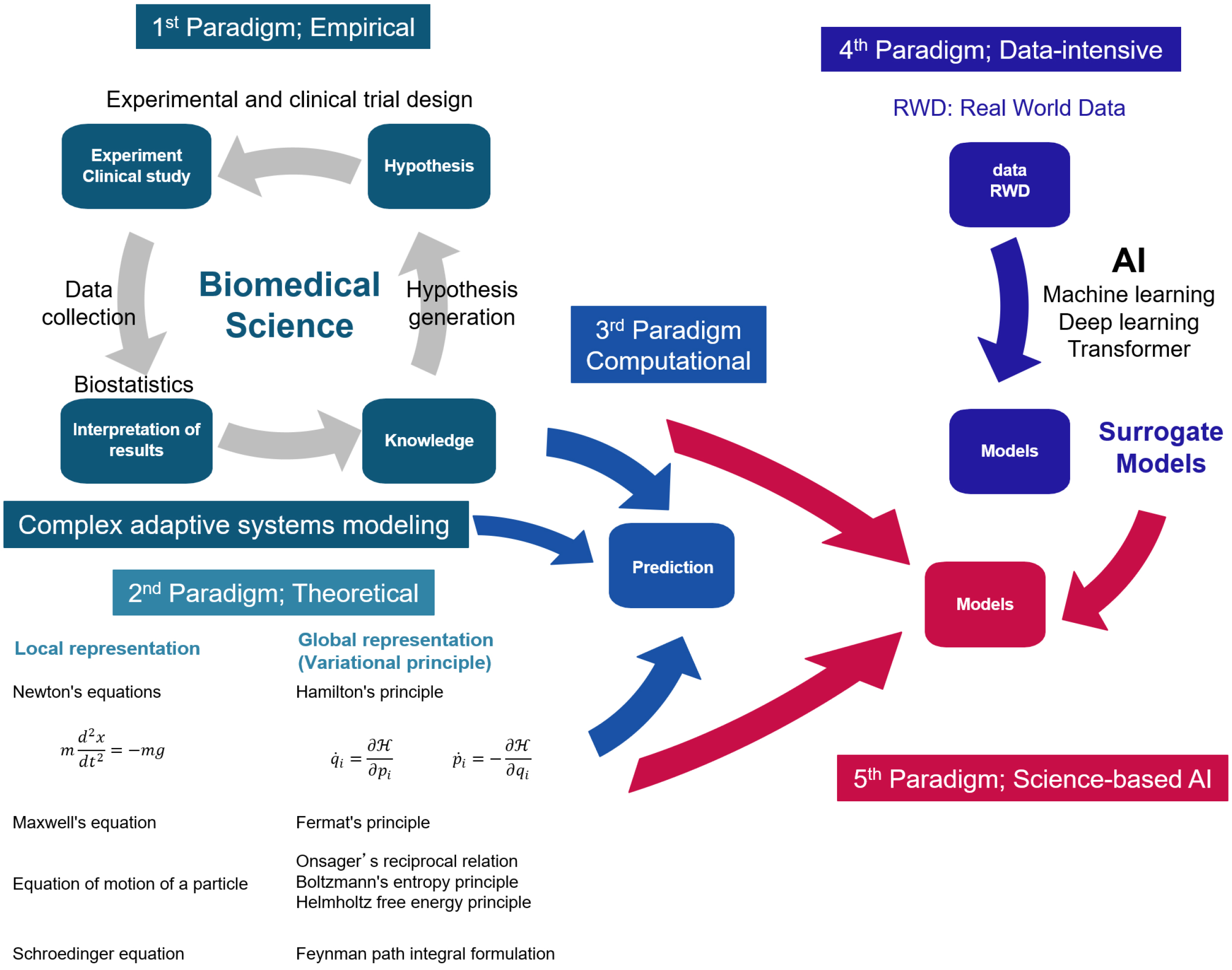
Figure 5. Fusion of causal inference and AI/ML
In medicine, a group of patients with the same symptoms is considered a homogeneous Gaussian population, and the hypothesis of a causal mechanism is tested by clinical statistics. However, patients with the same symptoms often exhibit diversity in other characteristics. If this diversity is not taken into account in hypothesis testing, the causal hypothesis may not be adequately reproduced. To solve this problem, it is effective to use ML to classify patients with the same symptoms into clusters and then test the causal mechanism. This is called “AI for Medical Science.”
From: Medical AI and AI for Medical Sciences
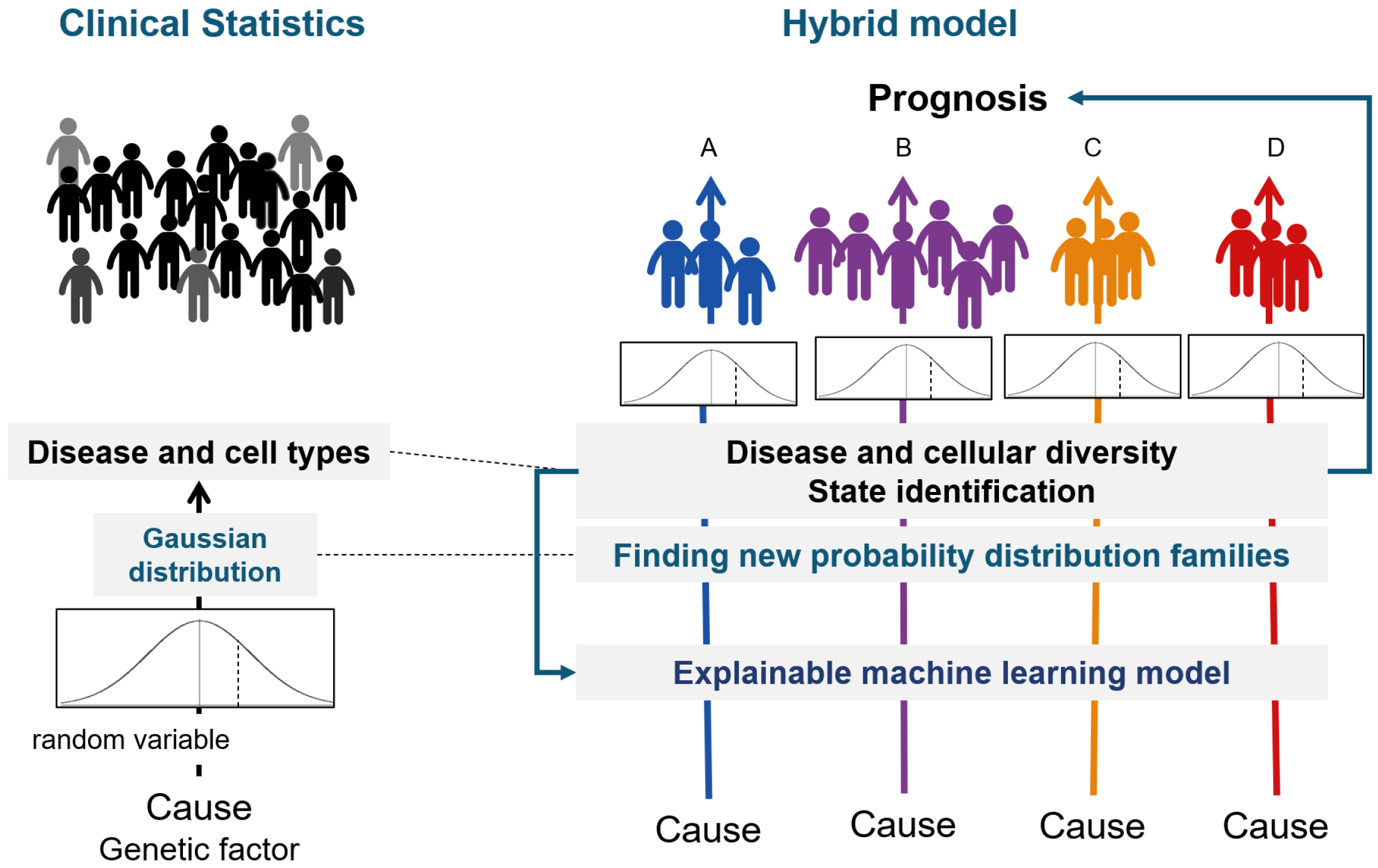
Figure 6. Standardization for life course inference
A quantitative representation of the process by which a person undergoes disease progression as gradual transitions of physical states can numerically express clinical symptoms, recovery, or chronic phase after treatment. This approach is essential to realize preventive medicine based on highly accurate prediction. To achieve this, it is effective to represent a person as a state rather than a symptom and to represent state changes as trajectories in a state space.
From: Medical AI and AI for Medical Sciences
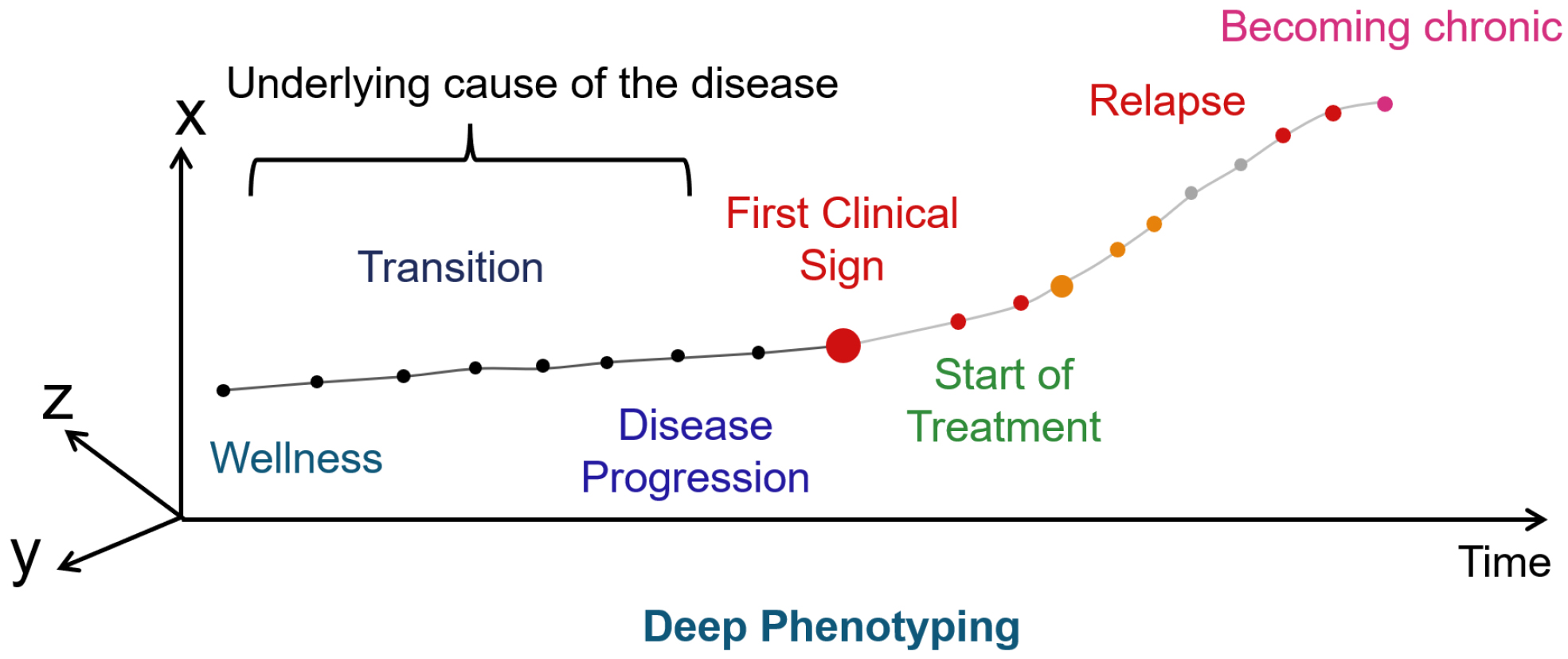
Figure 7. Large-scale disease model
The challenge for medicine is to adequately predict and control changes in a person’s physical state. (A) There are five main ways to conceptualize such changes: (1) causal mechanisms; (2) simulation using governing equations; (3) probabilistic models using ML; (4) information theory; and (5) physical theory. To build a reproducible, reliable, and unified concept of diseases, it is necessary to develop a disease model that integrates these five lines of reasoning. (B) The final large language model of medicine will be a collection of all individual disease models that integrate the five inferences. Since all models share tokens (physical states) and contexts (state transitions), the models can be integrated using transformers. The reliability of the inferences will not only be parameterized based on data, but also physical principles will be introduced.
From: Medical AI and AI for Medical Sciences
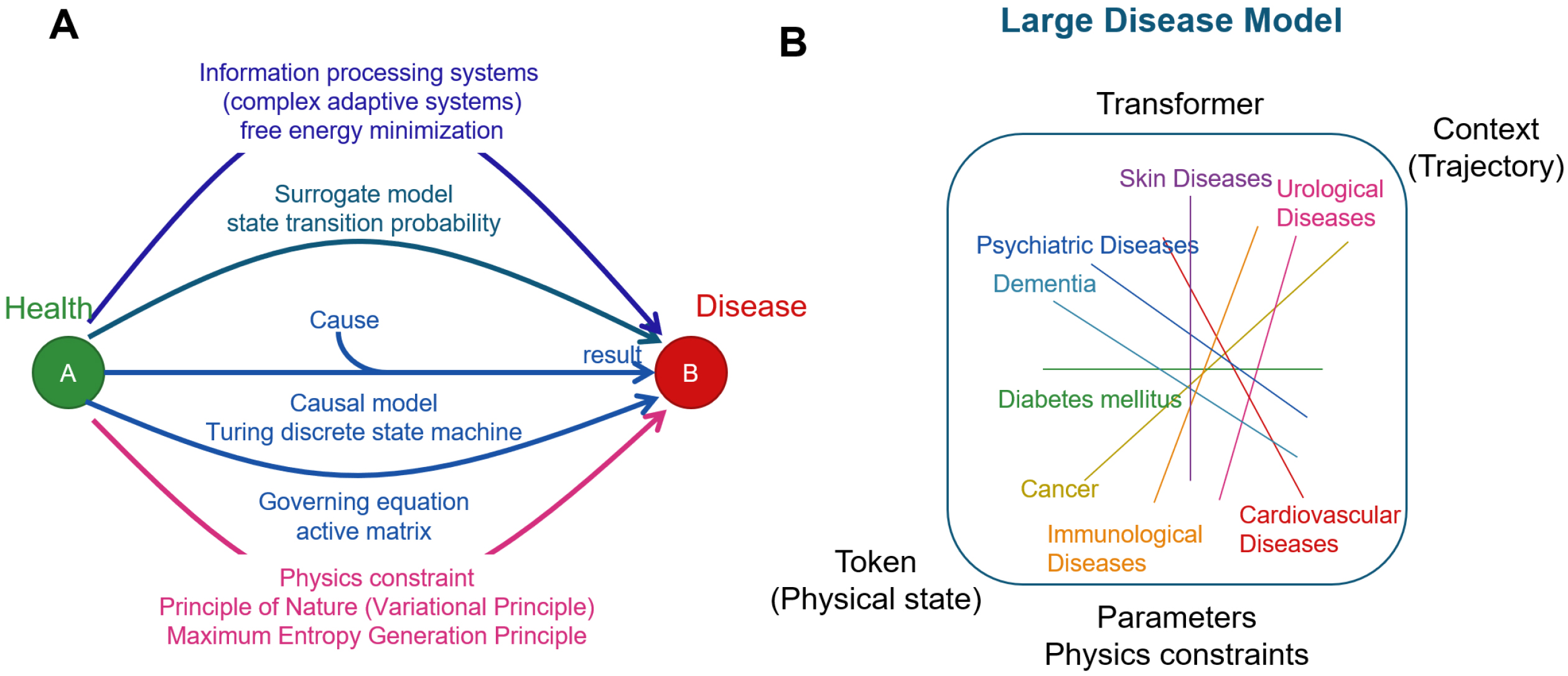